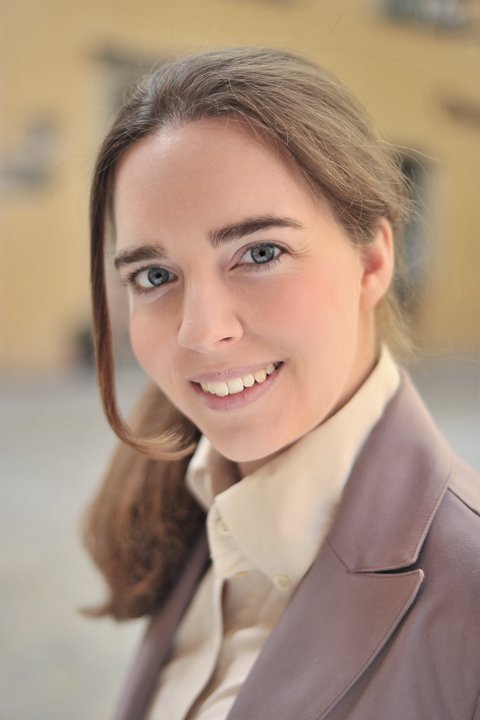
Dr. rer. nat. Helena U. Zacharias is heading the junior research group Computational biomarker discovery at the Department of Psychiatry and Psychotherapy, University Medicine Greifswald. After finishing her B.Sc. and M.Sc. in physics at the University of Regensburg, she acquired her Ph.D. in biology at the Institute of Functional Genomics, University of Regensburg. She conducted her postdoctoral studies at the chemistry department of the Ohio State University and at the Institute of Computational Biology, Helmholtz Center Munich.
Since 2019, she coordinates the e:Med junior research alliance CKDNapp, which develops a clinical decision support software to help the practicing nephrologist in personalized chronic kidney disease patient care. Helena’s research focusses on the improved prediction of adverse events in chronic kidney disease patients, and on obtaining a comprehensive understanding of metabolic pathomechanisms underlying this complex disease.
In her subproject, Helena will develop the mathematical models that enter CKDNapp. This includes
- designing risk scores to predict adverse events in patients with chronic kidney disease (CKD),
- establishing classifiers for CKD staging refinement,
- generating metabolic models and facilitate/verify their use by routine diagnostic assays,
- providing comprehensive mathematical modeling of CKD by mixed graphical models (MGMs) taking into account the complex interplay between all patient parameters, and
- enriching CKDNapp models with time-course information. These CKDNapp models will be calculated based on different biomedical and demographic variables, as well as untargeteted metabolomics data of the German Chronic Kidney Disease (GCKD) study employing state-of-the-art machine learning methods.
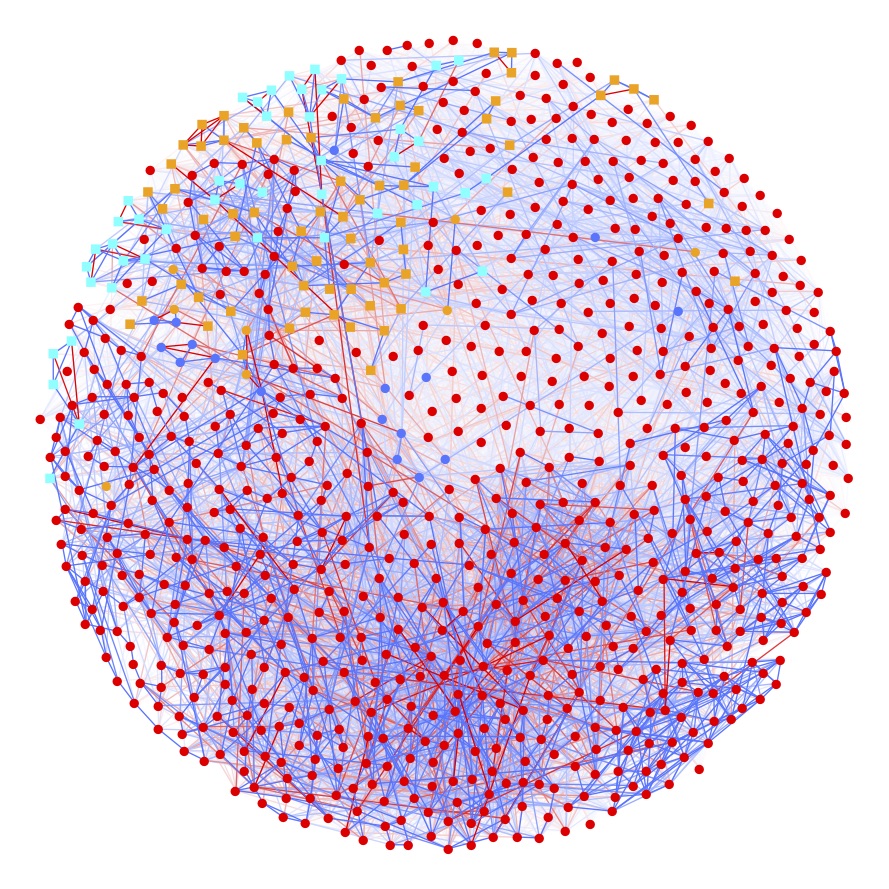
Network illustration of a mixed graphical model, which uncovers statistical associations between different variables measured within the GCKD study.
Circles or rectangles represent individual demographic, drug treatment, clinical chemistry, and metabolic variables, edges between two nodes represent an association between these respective variables.